
The observations in the sample are then split into g groups (we come back to choice of g later) according to their predicted probabilities. Specifically, based on the estimated parameter values, for each observation in the sample the probability that is calculated, based on each observation’s covariate values: The Hosmer-Lemeshow goodness of fit test is based on dividing the sample up according to their predicted probabilities, or risks. We will write for the maximum likelihood estimates of the parameters. In R this is performed by the glm (generalized linear model) function, which is part of the core stats library. The unknown model parameters are ordinarily estimated by maximum likelihood. The logistic regression model assumes that
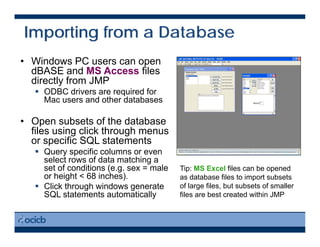
We will assume we have binary outcome and covariates. In this post we’ll look at the popular, but sometimes criticized, Hosmer-Lemeshow goodness of fit test for logistic regression. For binary outcomes logistic regression is the most popular modelling approach. That is, that the data do not conflict with assumptions made by the model.

Before a model is relied upon to draw conclusions or predict future outcomes, we should check, as far as possible, that the model we have assumed is correctly specified.
